Our AI supercomputer will be world's fastest this year: Meta
Meta (formerly Facebook) has built an AI supercomputer that, it claims, will be the fastest in the world when it's fully ready in mid-2022.
image for illustrative purpose
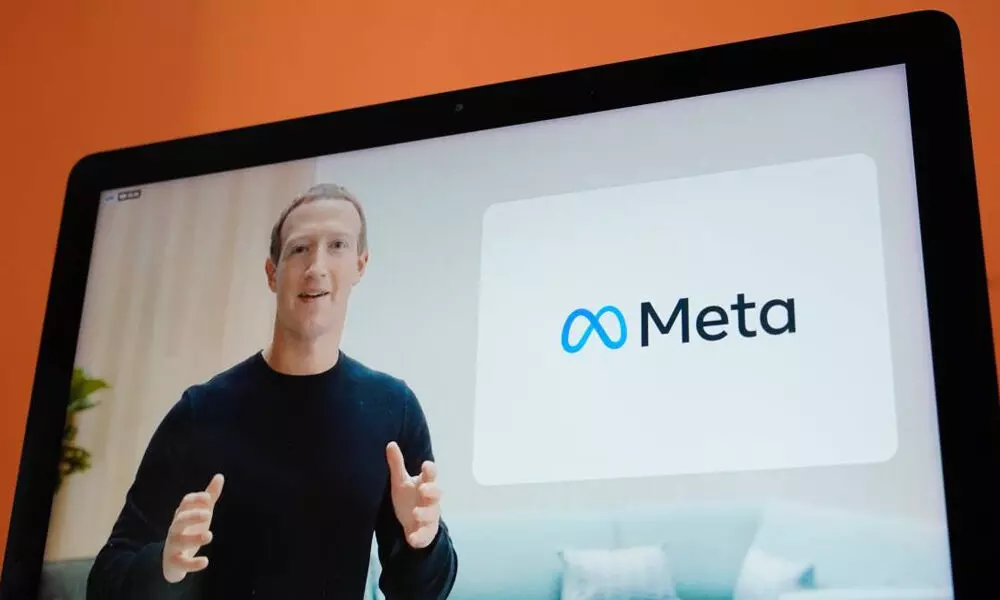
San Francisco, Jan 25 Meta (formerly Facebook) has built an AI supercomputer that, it claims, will be the fastest in the world when it's fully ready in mid-2022.
Called the AI Research SuperCluster (RSC), the machine is already being used by Meta researchers to train large models in natural language processing (NLP) and computer vision for research, with the aim of training models with trillions of parameters in the near future.
"RSC will help Meta's AI researchers build new and better AI models that can learn from trillions of examples; work across hundreds of different languages; seamlessly analyse text, images, and video together; develop new augmented reality tools; and much more," Meta engineers Kevin Lee and Shubho Sengupta said in a statement late on Monday.
The first generation of this infrastructure, designed in 2017, has 22,000 NVIDIA V100 Tensor Core GPUs in a single cluster that performs 35,000 training jobs a day.
"We wanted this infrastructure to be able to train models with more than a trillion parameters on data sets as large as an exabyte -- which, to provide a sense of scale, is the equivalent of 36,000 years of high-quality video," said Meta researchers.
Early benchmarks on RSC, compared with Meta's legacy production and research infrastructure, have shown that it runs computer vision workflows up to 20 times faster and trains large-scale NLP models three times faster.
That means a model with tens of billions of parameters can finish training in three weeks, compared with nine weeks before.
"RSC is up and running today, but its development is ongoing. Once we complete phase two of building out RSC, we believe it will be the fastest AI supercomputer in the world, performing at nearly 5 exaflops of mixed precision compute," said Meta.
Through 2022, Meta will work to increase the number of GPUs from 6,080 to 16,000, which will increase AI training performance by more than 2.5x.
The storage system will have a target delivery bandwidth of 16 TB/s and exabyte-scale capacity to meet increased demand, the company added.