Explainable AI Holds Key For Improving Trust Levels In The Machine Intelligence
AI already shaping critical decisions in finance, healthcare, law, and beyond. Yet, its opaque nature raises concerns about fairness, accountability, and trust
Explainable AI Holds Key For Improving Trust Levels In The Machine Intelligence
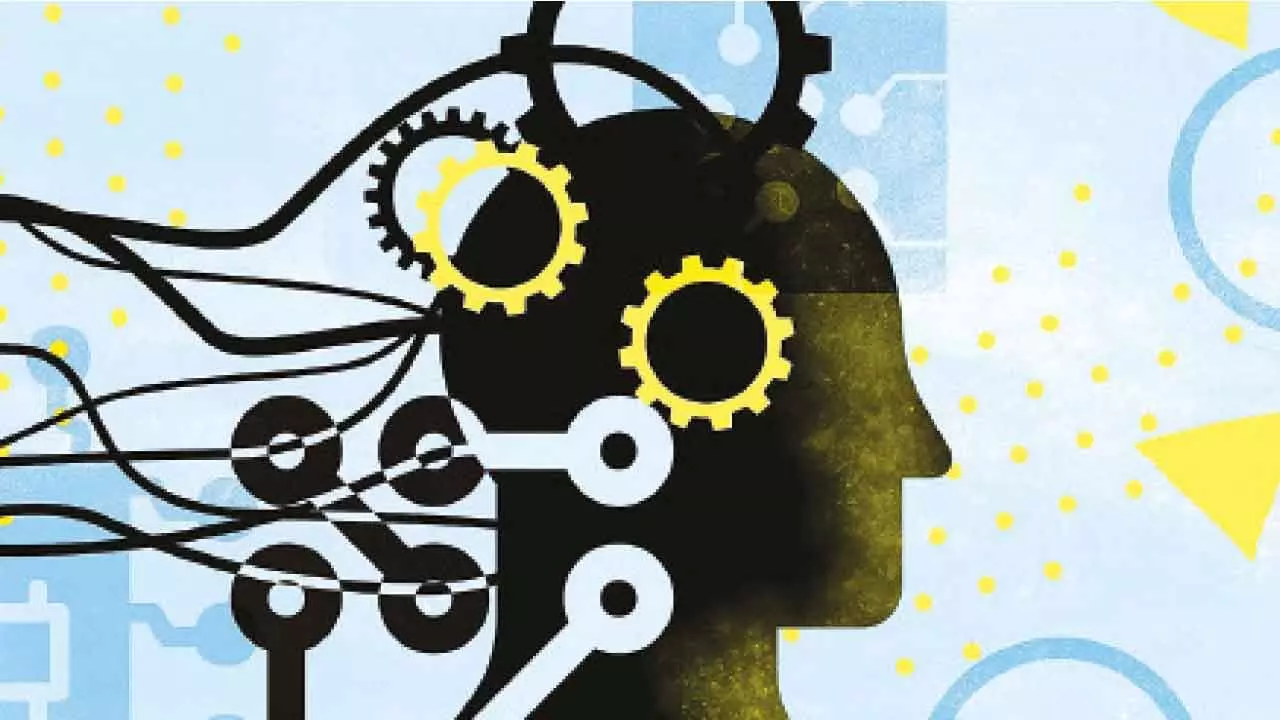
Explainable AI offers a solution, ensuring that AI decisions are not just accepted but understood. Without transparency, AI risks reinforcing biases and eroding public confidence. The future of AI must prioritize explainability, turning black boxes into glass boxes. After all, if humans are expected to justify their judgments, why should AI be any different?
Envision a world where artificial intelligence (AI) influences crucial moments in our daily lives - such as deciding who gets a loan or which job candidate is selected. This isn't a distant future; it's our reality today. AI powers many aspects of life, from autocorrect on smartphones to personalized Netflix recommendations, seamlessly integrated into how we live and work.
Yet, this reliance on AI raises concerns, as many decisions appear to come from a mysterious source, prompting the question—why? This is the "black box" of AI, a complex concept hidden in intricate algorithms. Explainable AI (XAI) steps in to help us understand how these systems function.
At its core, Explainable AI (XAI) encompasses a variety of techniques and methods aimed at helping humans understand and trust the outcomes of AI models. XAI offers insights into how an AI system arrives at its conclusions. By providing clear explanations, it promotes transparency and makes AI results more comprehensible for the users.
The Mystery of the Black Box
Let's say you apply for a loan. You've got a stable job, and a decent credit history, yet—boom! — your application gets rejected. You ask why, and the bank says, "Sorry, our AI system refused you" - End of the story.
But what if the AI rejected you because its training data mistakenly linked your PIN code with high default rates? Or it found some pattern in past approvals that was unrelated to your financial responsibility? Without transparency, there's no way for you to know.
XAI pulls back the curtain on AI decisions, making them understandable for humans. It's like turning a black box into a glass box, where you can see inside and understand what's happening. The implications of a world where AI drives decision-making without transparency could be severe.
Why Should You Care?
AI isn't just deciding who gets a loan; it also influences medical treatments, and how self-driving cars respond in emergencies. If we can't understand how AI reaches its conclusions, how can we trust it? This issue goes beyond convenience—it's about fairness and ensuring humans remain in control of crucial decisions. The more we depend on AI, the more we need clear explanations for its choices. If we wouldn't trust a judge who won't explain their verdict, why accept the same from AI?
As governments and corporations adopt AI-driven policies, a lack of transparency could lead to confusion and social unrest. If people don't trust AI's decisions, they will resist its adoption, slowing down beneficial technological progress.
Meet the "Glass Box" AI
So, how do we get from black boxes to transparent, explainable AI? Engineers have come up with creative ways to make AI explain itself. Here are some of the coolest methods:
SHAP (Shapley Additive Explanations) – Think of this like a scoreboard that shows which factors influenced a decision the most.
LIME (Local Interpretable Model-agnostic Explanations) – This technique creates a simpler, easier-to-understand version of the AI to explain decisions in plain terms.
Decision Trees – A flowchart-like structure that maps out every step the AI took before reaching its conclusion, making it as clear as a "choose-your-own-adventure" book.
These tools help AI talk back and answer the ever-important question: Why did you make that choice?
AI That Explains Itself: Real-Life Superpowers
Let's look at some ways explainable AI is already making a difference:
Healthcare: Doctors use AI to detect diseases like cancer from X-rays, but without explainability, they can't trust why the AI flagged a tumour. XAI can show which areas in the image influenced its decision, helping doctors make more informed choices.
Self-Driving Cars: Ever wonder how Teslas and Waymos decide whether to brake, speed up, or swerve? Explainable AI helps engineers debug and refine these decisions, making autonomous cars safer.
Fraud Detection: Banks use AI to detect credit card fraud, but false alarms are a big problem. If AI mistakenly flags your transaction as fraud, XAI can help the bank understand why, reducing unnecessary account freezes.
The Courtroom: AI is being used to predict crime risk in some legal systems. Without explainability, these systems could reinforce biases rather than fix them. XAI helps ensure justice is truly just.
Hiring Decisions: Many companies use AI to screen job applications. Without XAI, there's a risk that biases in training data could lead to unfair hiring practices. Imagine if an AI model learned that people with non-traditional names are less likely to get hired—without an explanation, this bias would go unchecked!
Recent success of DeepSeek-R1 AI
One of DeepSeek-R1's success secrets was its innovative focus on explainable AI. Competitors like OpenAI's o1 and Claude 3.5 prioritize performance and conversational fluency, while DeepSeek emphasizes transparency, allowing it to "think aloud" before giving answers.Which gave users some sense of logic behind the output. By prioritizing explainability, DeepSeek challenged the industry's old ways of size and opacity. In response both OpenAI's ChatGPT and Anthropic's Claude have improved transparency in their latest models.
The Future: AI We Can Trust
AI isn't going away—it's only becoming more powerful. The question is whether we build AI that's transparent and accountable, or whether we let black-box systems make decisions that no one understands. The goal of explainable AI isn't just to make machines less mysterious, but to make them more reliable, more ethical, and more aligned with human values. Imagine a future where AI works with us, instead of making us scratch our heads in confusion.
So, the next time AI decides something important for you - whether it's your loan application, your job interview, or even your healthcare - ask yourself: Does this AI owe me an explanation? Because the answer should always be yes.