AI vs. Machine Learning: Understanding the key differences
AI and Machine Learning are related but distinct: AI mimics human intelligence, while Machine Learning is a subset that enables systems to learn from data.
AI vs. Machine Learning: Understanding the key differences
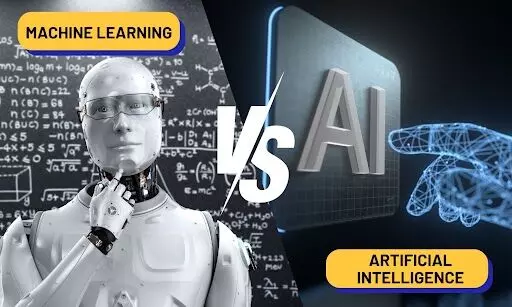
AI vs. Machine Learning: How Do They Differ?
Netflix knows what show you'll enjoy next, and Amazon suggests products you might want to buy because they know your preferences. But how?
Amazon and Netflix use Artificial Intelligence (AI) and ML to make your shopping and binge watching experiences more personal for you. Think of AI as the store manager who makes sure everything runs smoothly, from keeping track of stock to arranging products in the best way. ML is like the helpful assistant who watches what you look at and suggests things you might like, making your shopping easier and more enjoyable.
Let’s explore AI and ML and how they differ or complement each other. Imagine you're a chef stepping into a kitchen where two master chefs are working together—one is AI, and the other is ML. Both are highly skilled, but each has its own strengths.
AI is like the head chef, overseeing the entire kitchen. It’s designed to think and act like a human, making decisions, solving problems, and handling various tasks. Whether it's voice assistants like Siri or Alexa or self-driving cars, AI is everywhere. It’s like a chef who knows all the recipes and can create a dish by following specific rules or using intuition.
ML, on the other hand, is like the sous-chef, specializing in a specific skill and improving it over time. Instead of just following recipes, ML learns from each dish it makes, getting better with every try. It’s like training a junior chef by showing them how to cook a meal and letting them practice until they perfect it.
Roots in computer science AI and ML are deeply connected to computer science. They both use algorithms and data to not only mimic human intelligence but also to create systems that can learn on their own. They go beyond just copying human abilities like learning and problem-solving by analyzing data and getting better over time without needing human help. This overlap can be seen in real-world applications, like AI-powered chatbots and ML-driven predictive analytics in businesses.
Used in Many Industries AI and ML are used in numerous industries, transforming how businesses work. For example, in healthcare, AI tools help doctors diagnose diseases more accurately. In finance, ML algorithms detect fraud and assist with stock trading. Retail businesses use AI to personalize shopping experiences, and manufacturers use AI and ML to make production more efficient.
AI vs. Machine Learning: Key differences
Now that we’ve looked at their similarities, let’s understand how AI and ML are different. Knowing their distinct purposes, techniques, applications, and system needs will help clarify their unique roles.
Purpose and goals AI aims to create machines that can perform tasks that usually require human intelligence. This includes making decisions, solving problems, and interacting with humans in natural ways, like through speech or written language. AI can even take on creative tasks, like composing music or generating art.
ML, however, focuses on creating algorithms that help machines learn from data and make accurate predictions. The goal of ML is to continuously improve performance by learning from new data and automatically building models to handle complex tasks.
Strategies and techniques AI uses various methods to achieve its goals, including rule-based systems and neural networks, which mimic human brain functions to process complex data. It also involves natural language processing to understand and respond to human language.
ML, in contrast, relies on data-driven techniques. It includes supervised learning, where algorithms learn from a known dataset, and unsupervised learning, where outcomes are unknown. ML also uses reinforcement learning, where algorithms learn based on rewards and penalties, similar to how humans learn through experiences.
Applications AI has a wide range of applications. It’s used in healthcare for robotic surgeries, in the automotive industry for self-driving cars, and in customer service for smart chatbots.
ML is typically used in specific, data-heavy tasks. For instance, in healthcare, ML predicts the likelihood of disease development by analyzing patient data. In sports, ML helps optimize athletes’ training and performance analysis.
System requirements AI systems require powerful computers to handle complex algorithms and large datasets. They also need advanced hardware like CPUs and GPUs for tasks like image and speech recognition.
ML systems, on the other hand, emphasize the need for strong data processing capabilities. They rely on specialized tools like TensorFlow or PyTorch, which are software libraries used to build and run complex ML models.
How AI and Machine Learning are used in different industries
AI and ML are being used across many industries to improve processes, boost efficiency, and enhance customer experiences. Here are some examples:
AI in manufacturing
- Predictive maintenance: AI predicts when equipment might fail, helping reduce downtime. Siemens uses sensors and data analysis to monitor machine health, which lowers maintenance costs.
- Quality control: AI systems inspect products for defects. For example, BMW uses AI to improve its manufacturing processes.
- Supply chain management: Bosch uses AI to predict demand, manage inventory, and optimize logistics routes, making the supply chain more efficient.
AI and ML in banking
- Fraud detection: HSBC uses AI to detect fraudulent transactions by analyzing patterns in real time, enhancing security.
- Customer service bots: Bank of America’s chatbot, Erica, provides personalized banking advice and helps customers manage their finances.
- Credit scoring: Lending Club uses ML to create more accurate credit scores, offering better loan options.
AI in healthcare
- Diagnostic assistance: Google Health’s AI helps diagnose diseases from medical images, improving patient outcomes.
- Patient care optimization: AI provides personalized treatment plans based on patient data, adjusting treatments in real time.
- Personalized medicine: Companies like 23andMe use AI to analyze genetic data, helping tailor healthcare to individual needs.
AI in industrial production
- Automated quality control: Tesla uses AI to ensure high product quality by analyzing production data in real-time.
- Energy efficiency: AI in Tesla’s factories optimizes energy use, reducing costs and supporting sustainability.
- Robotics and automation: Fanuc’s AI-driven robots can learn and adapt to new tasks, increasing production flexibility.
AI in retail
- Personalized recommendations: Alibaba uses ML to analyze customer data and provide personalized shopping suggestions.
- Stock management: Target’s AI system manages inventory based on real-time sales data and trends.
- Customer behavior analysis: Nordstrom uses AI to understand shopping patterns, improving marketing strategies and store layouts.AI and machine learning are closely related, yet they have distinct purposes. AI aims to broaden what machines can do by mimicking human intelligence, while ML, as a subset of AI, focuses on teaching machines to learn from data and make smart decisions. As these technologies continue to grow, they will play key roles in shaping the future across various industries.